January 16, 2024
【Case study】Ultra Large Scale Virtual Screening
Discovery of novel compounds with human Aryl hydrocarbon receptor (AhR) antagonist activity
We would like to introduce a case study of a domestic pharmaceutical company’s search for novel hAhR inhibitors using both Structure Based and Ligand Based computational approaches including AI and ML methods to screen a compound library of several hundred million to several billion compounds.
Study preparation
Following establishment of an NDA with the client, Xeureka conducted an initial investigation of relevant patents, publications, and other literature, as well as a simple feasibility study, and based on these results proposed a few potential process flow patterns that we considered effective to address the project goals, together with a quotation. (Free of charge up to this point.)
【Receptor】
Crystal structure of hAhR is unknown and hAhR PAS-B domain (ligand binding site) is a flexible protein.
・Obtain the crystal structure of the protein appeared difficult
・The protein flexibility allows it recognize a variety of different compounds
・The protein structure (holoform) is different for each ligands
・The target was considered difficult for SBVS (Structure Based Virtual Screening)
【Ligand】
Compounds with reported activity toward AhR: around 14,000 (Fig. 1)
After extracting only human agonist and antagonist data from the above and checking their validity, corrections were made for 4 patents and 2 papers among the referenced citations, and a validated data set created.
・Agonists: approx. 700 compounds
・Antagonists: approx. 3600 compounds
・Agonist/antagonist: approx. 800 compounds
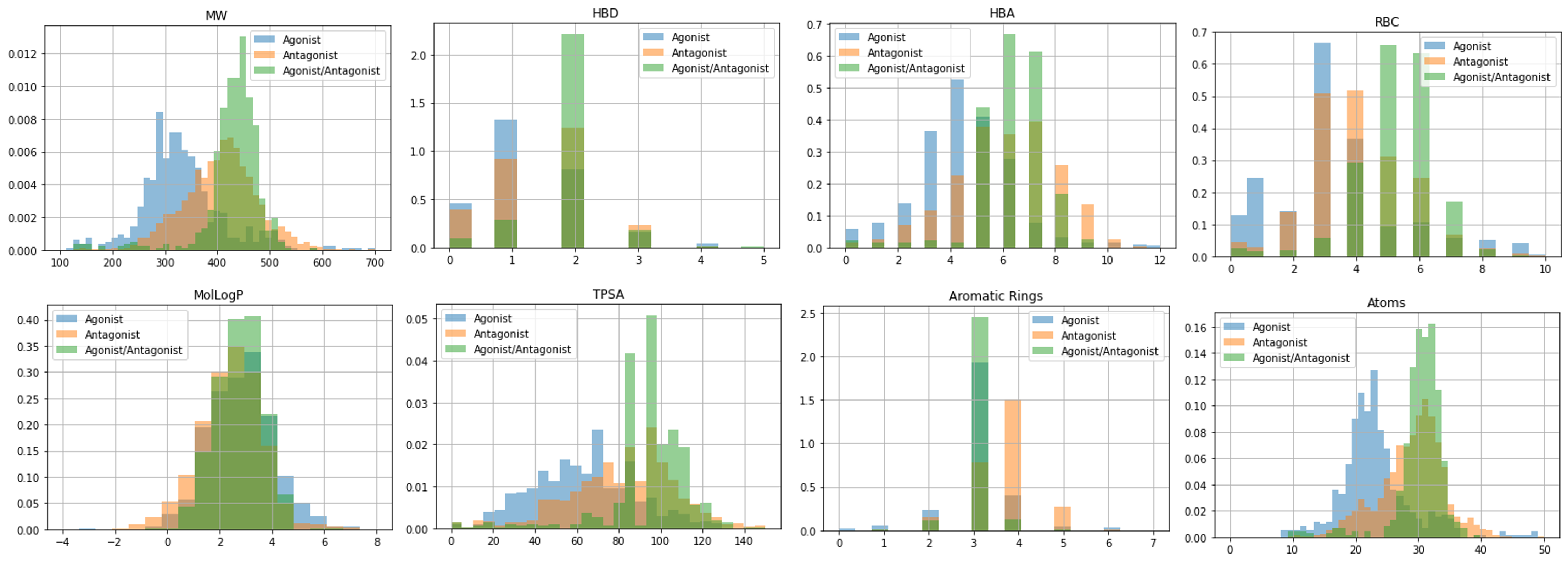
Fig 1. Chemical property distributions for known agonist and antagonist compounds(■:Agonist, ■:Antagonist, ■:Agonist/Antagonist)
Screening flow
Based on the information from the preliminary study, we proposed several screening flows and finally decided to proceed with hit discovery for the project using both Ligand Based Virtual Screening (LBVS) and Structure Based Virtual Screening (SBVS). The process used for these studies is shown below (Fig. 2), with activity for the new compounds confirmed by synthesis and assay.
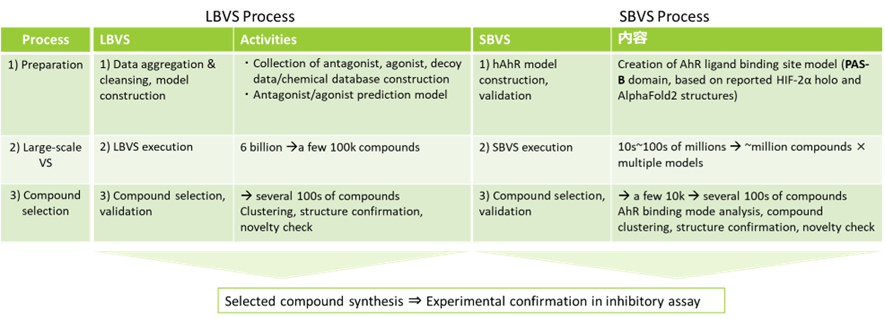
Fig 2. Outline of project process
LBVS process
In this project, since a large number of known antagonists consisting of multiple chemotypes have been reported, we created a machine learning antagonist discrimination model that utilizes these chemotypes to screen for new chemical matter.
【Creation of training data set】
Active data were mostly collected from the data confirmed in the preliminary survey above. As part of our data quality control, Xeureka checked the patents and papers that serve as the data sources to ensure that there were no problems with the activity information. Even when data is obtained from curated, commercial databases, it is often incorrectly annotated due to errors in data registration. We then checked the relationship between the activity values and chemical properties, diversity of chemotypes, etc., and confirmed that there were no problems in using the training data for creating an antagonist discrimination model (Fig. 3).
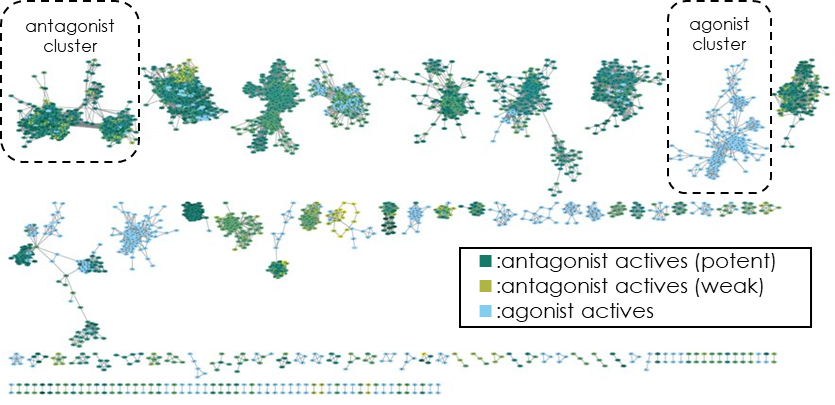
Fig 3. Analysis of cluster/chemotype diversity by chemical space network (expressing the relationship between compounds from the largest common substructure
At the same time, Decoy data to be used as the negative data set were collected from various databases and sources, referring to the distribution of chemical properties of the Active data collected. Finally, comparing the similarity distribution of the features used in the discriminant model with that of the Active data, we checked whether the data set would be capable of generating an effective discriminant model (Fig. 4).
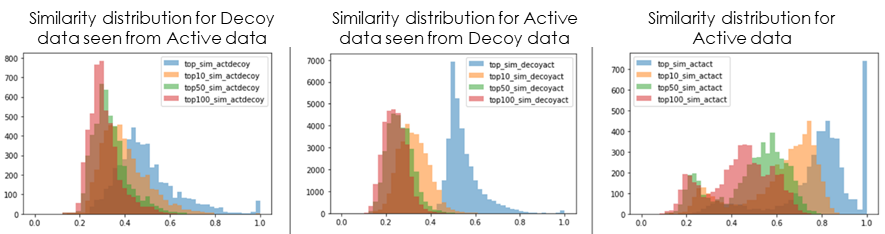
Fig 4. Similarity distribution of compounds between each dataset (■:maximum similarity, ■:top 10 percentile similarity, ■:top 50 percentile similarity, ■:top100 percentile similarity)
【Creation and screening of training model】
The training data set was created, in various cases including data splits and feature weights, learning algorithms, and hyperparameters for the learning algorithms (Fig. 5). Generally, models were then selected based on various the performance indicators of the predictive models. However, when conducting large-scale screening, there are differences in models that are difficult to discern using machine learning performance indicators.
In some cases, Xeureka performs prescreening of an ultra-large library using a diverse subset of that library in a model that has been narrowed down to a certain level based on performance indicators. For this project, a library of approximately 6 billion compounds was to be screened, and as part of the pre-screening, a diverse set of approximately 40 million compounds was screened. Based on the results of prescreening each model, those models that met the objectives of the project were selected. In this project, the intention was to obtain hits for as many different chemotypes as possible, while limiting the number of compounds to be synthesized, so the strategy was to select multiple models that were less likely to generate false positives to cover chemical space efficiently.
After that, large–scale screening was conducted using the models selected, and a list of several thousand compounds was created, as well as the list of indicators narrowed down. Finally, a list of approximately 200 candidate compounds was created after inspection by medicinal chemists for novelty, feasibility for conducting SAR studies by chemical optimization, and other structural features.
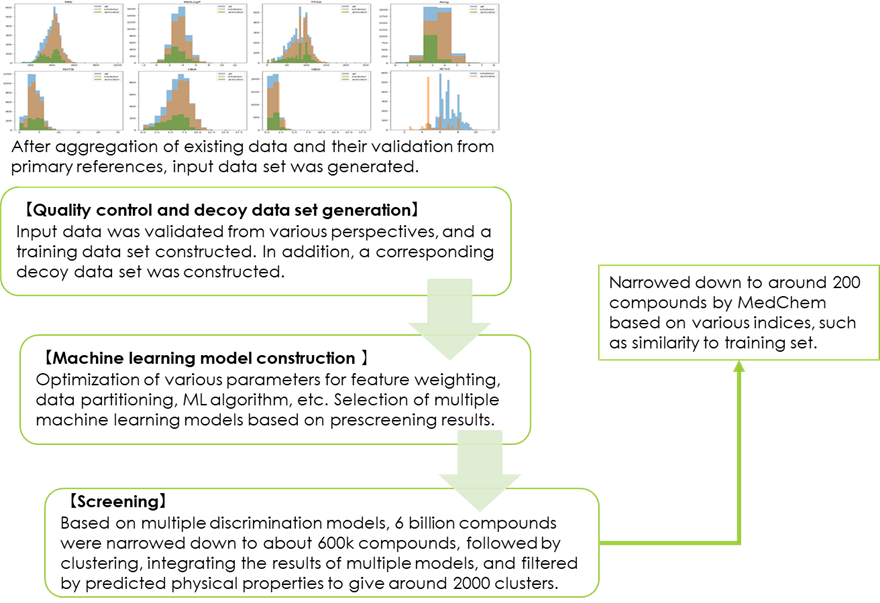
Fig 5. LBVS process flow diagram
SBVS process
As mentioned above, the structure of the target protein hAhR is expected to change depending on the structure of its ligand, making a structure-based approach highly challenging. In this project, we created several complex models that fit several known representative ligand chemotypes, screened each of them, and merged the results.
【Construction of protein 3D structural model】
As a starting point, we began by constructing a three-dimensional structural model of the target protein, as the crystal structure of an hAhR-small molecule ligand complex had not yet been obtained (at the time of project implementation). Based on the approach outlined above, we decided to select a model according to the following process.
・Protein structure is divided into different classes, and multiple representative models prepared.
・Evaluation of each model is conducted based on whether the binding of known antagonists can be predicted by docking.
We initially created 6 models based on human HIF-2α and Drosophila AhR (PDB: 7VNH) as templates based on the literature (Nature Communications 13, 6234 (2022)), and optimized their structures by MD. Attempts to verify the accuracy of antagonist recognition by docking showed very low accuracy for all models. After analyzing these results and selecting certain complex structures and additional template structures such as those generated by AI, we selected multiple ligands for each chemotype from among the known antagonists and created models for a total of more than 100 template-ligand complexes. We performed structural relaxation (MD) on more than a dozen of these complexes, extracted snapshots based on several indices such as binding free energy, and finally evaluated about 50 models by Docking (Fig. 6).
Fig 6. Example of performance evaluation of the 3D structural model generated. The vertical axis in the lefthand figures shows the number of compounds, and the horizontal axis shows the docking score (the further left, the better). In the re-created 3D structure model, even with mixed agonist and decoy data, agonist compounds are clustered at the top of the docking score, and the model can recognize agonist compounds by docking. The righthand figures show the AUC for each of the left figures, which represents the accuracy of antagonist recognition by docking.
【Screening】
From the models created, four models were finally selected and screened according to the flow outlined in the figure below for each model (Fig. 7).
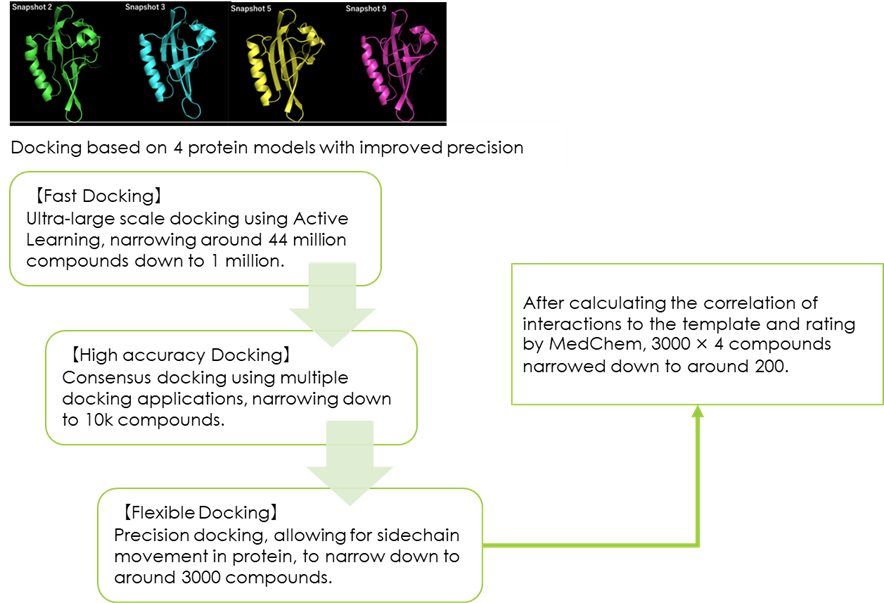
Fig 7. SBVS process flow diagram
Synthesis and assay results
Compounds selected from each LBVS and SBVS campaign were synthesized, with 129 compounds selected for LBVS and 109 compounds selected from SBVS tested for inhibitory activity against hAhR, with known antagonist compounds included for comparison as positive controls.
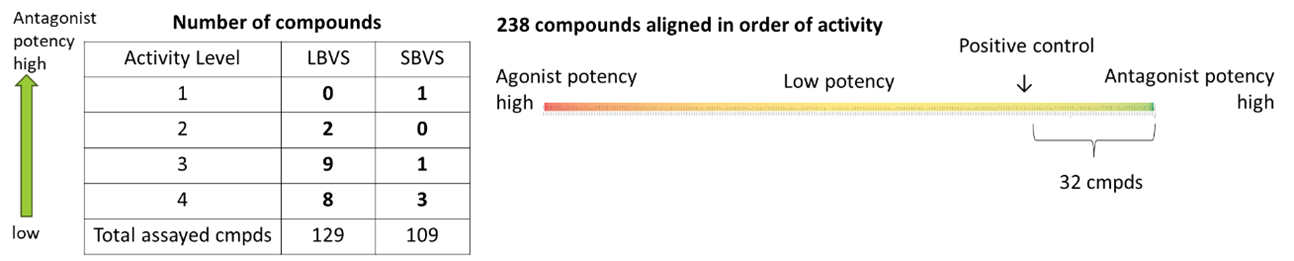
Fig 8. Summary of synthesis and assay results
A total of 32 compounds, including 25 compounds (7 scaffolds) selected from the LBVS results and 7 compounds (5 scaffolds) selected from the SBVS results, showed stronger inhibitory activity than the positive control, indicating an efficient level of hit identification for various scaffolds with activity in the single-digit μM range.
In summary
Xeureka supports your drug discovery research from the initial hit identification proposal, as well as the hit to lead and lead optimization stages. We provide free–of–charge proposals, including feasibility studies and various types of in silico discovery methods. We are also available for consultation on more focused or individual processes such as protein structure generation only or construction of models for ADME property prediction only. For any questions, please contact us from the contact page below!
CONTACT | Xeureka, Inc. (xeureka.co.jp)